Extensions to previous packages for quick and easy analysis of large (big data) sets and the creation of predictive models using machine learning, AI and ETL.
View Full Version Modeler use Statistica primarily for the analysis of large (big data) sets and for the creation of advanced predictive models. The package includes a range of machine learning, AI and ETL tools.
The Modeler version will be used primarily by data scientists and analysts for predicting and modelling the behaviour of variables under different conditions.
The application is available in desktop, network and server form.
No need to enter payment details
Import of data
Modeler is fully compatible with xlsx (including xls), csv and fixed width data (e.g. in text files). It will allow you to:
- retrieve data from SQL, NoSQL and other databases,
- via integrated PI connector retrieve data from OSIsoft PI system (a popular solution for operational data management),
- import Spotfire SBDF data files,
- integrate two or more data sets into one graphical environment and a series of outputs.
Data preparation
Modeler offers automated data cleaning from duplicate, inconsistent and outlying values (or their recoding) using the so-called Data Health Check (DHC) function.
For advanced data transformation, the tool Rules Builderwhich allows you to process data from different sources according to complex rules (even using conditional expressions).
For easier processing, bring your data closer to a normal layout by using the built-in Box-Cox transformation.
Data evaluation
In the Modeler version, you can evaluate measured data (including big data files), among others using:
- classical methods descriptive, parametric and non-parametric statistics,
- exploratory analysis and visualization,
- multivariate statistical methods for data organization and classification,
- advanced linear and non-linear models,
- estimation of many variance components and accuracy in the data sets (Variance Estimation and Precision).
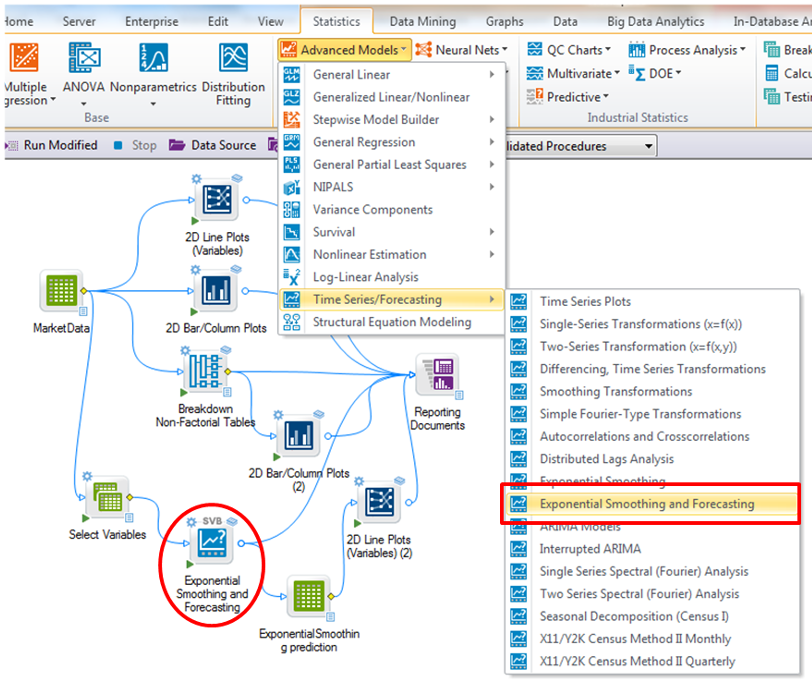
Predictive modelling
Use data mining, text mining and neural network tools to create models of the behaviour of the observed variables in different situations.
The modern PMML language is used to generate them. Outputs can be further modified as required.
Other features
Statistica in this version also offers the possibility to program custom scripts in R, Python or C#. The modeler can also be used for e.g. for:
- understanding the key parameters affecting critical quality attributes (process analysis, quality control and multivariate statistical process control functions),
- design of experiments and their virtual execution (design of experiments function – Design of Experiments, test power analysis – Power Analysis and interval estimation – Interval Estimation).
Visualisation and outputs
In Modeler you can see the distribution of the acquired data and the results, among others in histogram, line, box, point, scatter and quantile plots and more frequently used 2D and 3D imaging methods.
The results obtained can export e.g. in the form of:
-
simple and advanced reports,
-
entry into different types of databases,
-
MS Word (docx), MS Excel (xlsx) and text files (csv) or pdf.
Overview of analytical functions
- ANOVA/MANOVA
- Association Rules
- Automated Neural Networks
- Boosted Tree
- Calculators; Distributions, Pearson Product Moment Correlation Coefficient, Six Sigma
- Canonical Analysis
- Classification Trees
- Cluster Analysis
- Correlation
- Correspondence Analysis
- Cox Proportional Hazards Models
- Data Miner Recipes
- Descriptive Statistics
- Design of Experiments (DOE)
- Discriminant Function Analysis
- Distribution Fitting
- Distributions & Simulation
- Dynamic Time Warping
- Extract, Transform, and Load(analytics are used to align time based data)
- Factor Analysis
- Faster Independent Component Analysis
- Feature Selection
- Fixed Nonlinear Regression
- General CHAID Models
- General Classification and Regression Trees (C&RT)
- General Discriminant Analysis (GDA)
- General Linear Models (GLM)
- General Partial Least Squares Models (PLS)
- General Regression Models (GRM)
- Generalized Additive Models (GAM)
- Generalized Linear/Nonlinear Models (GLZ)
- Goodness of Fit, Classification, Prediction
- Independent Component Analysis
- Interactive Tree (C&RT, CHAID)
- Lasso Regression
- Link Analysis
- Log-Linear Analysis of Frequency Tables
- Machine Learning (Bayesian, Support Vectors, K-Nearest)
- Multidimensional Scaling (MDS)
- Multivariate Adaptive Regression Splines (MARSplines)
- Multiple Regression
- Nonlinear Estimation
- Nonparametric Statistics
- Power Analysis and Interval Estimation
- Multivariate Statistical Process Control (MSPC – PCA/PLS)
- Optimal Binning
- Predictor Screening
- Principal Components & Classification Analysis (PCCA)
- Process Analysis
- Quality Control Charts
- Random Forests
- Rapid Deployment of Predictive Models (PMML)
- Reliability and Item Analysis
- Sequence and Link Analysis
- Stepwise Model Builder (what-if)
- Structural Equation Modeling and Path Analysis (SEPATH)
- Survival & Failure Time Analysis
- Time series / forecasting
- t-tests and other tests of group differences
- Tabulate
- Variance Components & Mixed Model ANOVA/ANCOVA
- Weight of Evidence